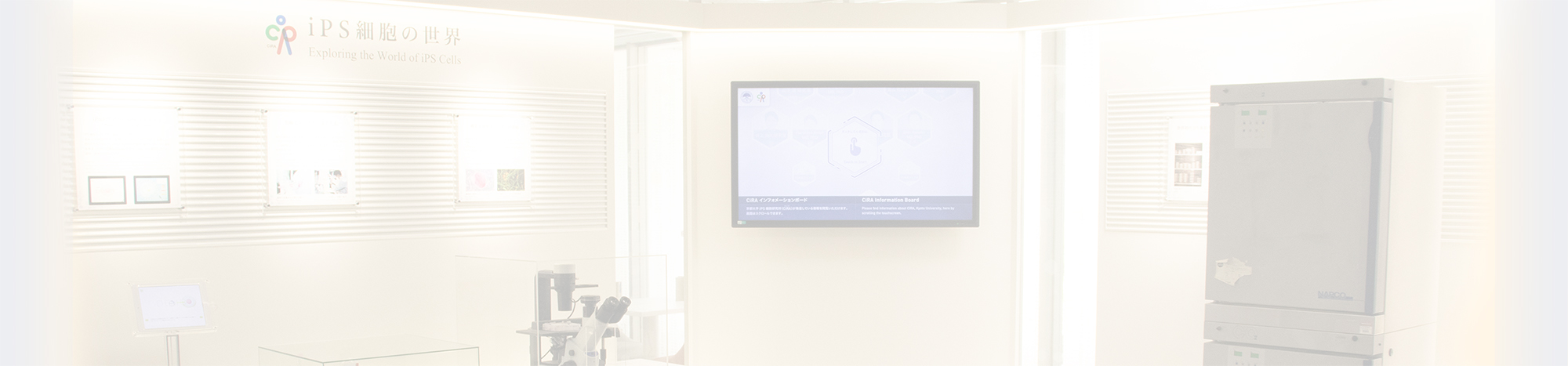
News & Events
News & Events
News
June 15, 2016
Machine learning measures drug toxicity
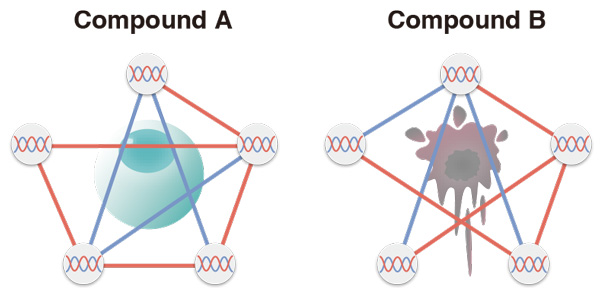
Different drug compounds activate different gene networks in a cell. Measuring the gene networks with machine learning provides almost 100% prediction accuracy on the toxicity of the experimental drug.
Before a drug reaches the market, it must go through vigorous testing to confirm both its efficacy and more importantly its safety. Conventionally, to evaluate an experimental drug, toxicologists rely on the quantitative structure-activity relationship (QSAR) model. However, too often, drugs that are deemed safe by QSAR in animal models fail in human ones.
The problem with QSAR, according to Professor Fujibuchi, is that it primarily considers the chemical structure and not the cell activities. "It does not consider the cell. If we switch the cell type, it should not work in many cases," he explained, adding that neglecting cell activity discounts the developmental stage of the cell.
This neglect could have important implications even if a drug passes human tests, since experimentally it is difficult to prepare cells that reflect the wide age groups the drug may serve in the general population.
To overcome these problems, the Fujibuchi lab shows in its latest publication in Nucleic Acids Research that machine learning may be more robust at evaluating toxic cellular effects.
Although gene expressions are one way to evaluate cell activity, the new method depends on constructing gene networks so that it further considers the interplay between genes. "We wanted to use Bayesian networks because it is more robust than gene expressions," said Fujibuchi.
The research team found that a Bayesian network consisting of just 10 genes was sufficient to predict the effects of a drug candidate. "Ten is kind of low," he said, "I am surprised."
The group tested its model on 20 drug compounds with known toxicological effects, findings its accuracy consistently scored over 97% compared with probabilities that dipped below 80% in some cases that only considered gene activities. Moreover, the results indicated that different information can be acquired by analyzing the gene expressions and networks separately, suggesting a combination of the two could provide more reliable testing.
Another key outcome of the study was that the small network of 10 genes significantly reduced the computation time for one test to mere minutes. Considering that each drug needs to be tested at multiple doses and multiple durations, which adds both cost and time to a toxicology test, Fujibuchi is hopeful that this Bayesian network approach will attract collaborations with industry. "I hope many pharmaceuticals will be interested in our work," he said.
Paper Details
- Journal: Nucleic Acids Research
- Title: Prediction of Developmental Chemical Toxicity based on Gene Networks of Human Embryonic Stem Cells
- Authors: Junko Yamane1,2, Sachiyo Aburatani3, Saoshi Imanishi2, Hiromi Akanuma4, Reiko Nagano4, Tsuyoshi Kato5, Hideko Sone4, Seiichiroh Ohsako2, Wataru Fujibuchi1
- Author Affiliations:
- Center for iPS Cell Research and Application (CiRA), Kyoto University, Kyoto, Japan
- Center for Disease Biology and Integrative Medicine, The University of Tokyo, Tokyo, Japan
- Computational Biology Research Center, Advanced Industrial Science and Technology (AIST), Tokyo, Japan
- Research Center for Environmental Risk, National Institute for Environmental Studies, Tsukuba, Japan
- Department of Computer Science, Gunma University, Gunma, Japan