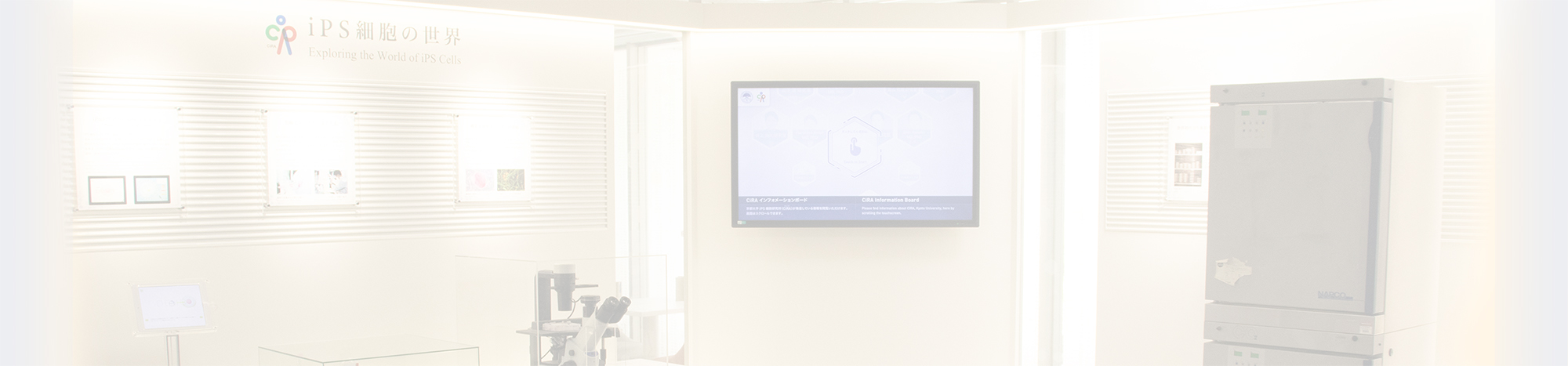
News & Events
News & Events
News
November 16, 2020
The heat diffusion equation shows its power in drug discovery for brain diseases
Drug discovery involves the investigation of a massive number of chemical compounds - typically in the millions. In recent years, machine learning has been applied to hasten the discovery and reduce the time and cost. Machine learning relies on mathematical algorithms, but current approaches are limited. A collaboration of research institutes led by Dr. Haruhisa Inoue, who serves as a professor at the Center for iPS Cell Research and Application (CiRA), Kyoto University, a principle investigator at T-CiRA, Takeda Pharmaceuticals, and a senior visiting scientist at the Riken Center for Advanced Intelligence Project) reports the first application ever of the heat diffusion equation in machine learning for drug discovery. With this new prediction model and motor neurons derived from patient induced pluripotent stem (iPS) cells, the researchers discover five new chemotypes that show promise for treating amyotrophic lateral sclerosis (ALS).
Drug screening can be broken down into two types. Target-based screenings seek chemical compounds that bind to a specific molecule, changing the function of the molecule. This approach is effective if researchers understand the role of the target molecule in the disease. However, for many neurological diseases like ALS, the vast majority of cases are sporadic.
"For sporadic diseases, the putative disease mechanism and genetic causes are diverse, therefore, it is not easy to know the focused target for the drug," explains Inoue.
Thus, there is a growing shift toward phenotypic drug screenings, in which the compounds act on a phenotype, such as heart rate, rather than on some molecular target. ALS patients are divided into familial and sporadic types. In the first group, a gene mutation is recognized as causing the disease, but in the second group, no one gene mutation is the cause. However, all ALS patients show one common phenotype - the death of motor neurons, which causes their muscles to die and leaves the patients unable to walk and ultimately unable to breathe.
Machine learning is already being applied to drug discovery, but phenotypic drug discovery has several unique challenges.
"The heat diffusion equation model has the advantage of being more flexible," says Dr. Yoshikazu Giga, one of the main developers of the model.
Fundamentally, the heat diffusion equation is a partial differential equation which describes how heat conducts and particles diffuse from one spot to another spot. It has many abstract applications, from quantum mechanics to financial markets. In medical research, it has been used to identify critical genes for a disease and to predict the side effects of a drug.
HDE model was developed by Takeda in collaboration with the univ. of Tokyo by applying heat diffusion equation. In the ALS study, Inoue and his team used this equation for machine learning to create a prediction model that could distinguish active and inactive compounds from the millions screened.
There is very little available in terms of treatment for ALS patients. There is no cure, and approved drugs prolong the patient's lifespan approximately three months at best, which is still impressive since a patient has only a fifty percent change living beyond two years of the diagnosis.
Inoue is an expert of iPS cell technology for medical applications. His team applied the new machine learning system by HDE model to evaluate millions of compounds that could suppress the death of motor neurons derived from ALS patient iPS cells. Among the hundreds of hits, several compounds with new chemotypes were found. When excluding compounds with known toxic effects, the new prediction model discovered new chemotypes that had never been identified previously.
"There are only two drugs with modest effects available for ALS. We need to study compounds with strong activity to find an effective new drug," says Inoue.
Notably, the five new chemotypes had no similarity with these two drugs. Cellular assays confirmed that the five protected the motor neurons, in most cases outperforming the two approved drugs.
ALS was selected because it is the disease of interest by the medical laboratories participating in the project. The researchers hope to partner with other groups working on diseases of other organs to demonstrate the versality of the heat diffusion equation in machine learning for drug discovery.
Paper Details
- Journal: Patterns
- Title: Prediction of Compound Bioactivities Using Heat-Diffusion Equation
- Authors: Tadashi Hidaka1, Keiko Imamura2,3,4,5, Takeshi Hioki1,3, Terufumi Takagi1, Yoshikazu Giga6,7, Mi-Ho Giga6,7, Yoshiteru Nishimura8, Yoshinobu Kawahara8,9, Saotru Hayashi1,3, Takeshi Niki2,3, Makoto Fushim1 and Haruhisa Inoue2,3,4,5
- Author Affiliations:
- Research, Takeda Pharmaceutical Company Limited, Fujisawa, Japan
- Center for iPS Cell Research and Application, Kyoto University, Kyoto, Japan
- Takeda-CiRA Joint Program (T-CiRA), Fujisawa, Japan
- iPSC-based Drug Discovery and Development Team, RIKEN BioResource Research Center BRC), Kyoto, Japan
- Medical-risk Avoidance based on iPS Cells Team, RIKEN Center for Advanced Intelligence Project (AIP), Kyoto, Japan
- Graduate School of Mathematical Sciences, University of Tokyo, Japan
- Institute for Mathematics in Advanced Interdisciplinary Study, Sapporo, Japan
- Structured Learning Team, RIKEN Center for Advanced Intelligence Project (AIP), Fukuoka, Japan
- Institute of Mathematics for Industry, Kyushu University, Fukuoka, Japan