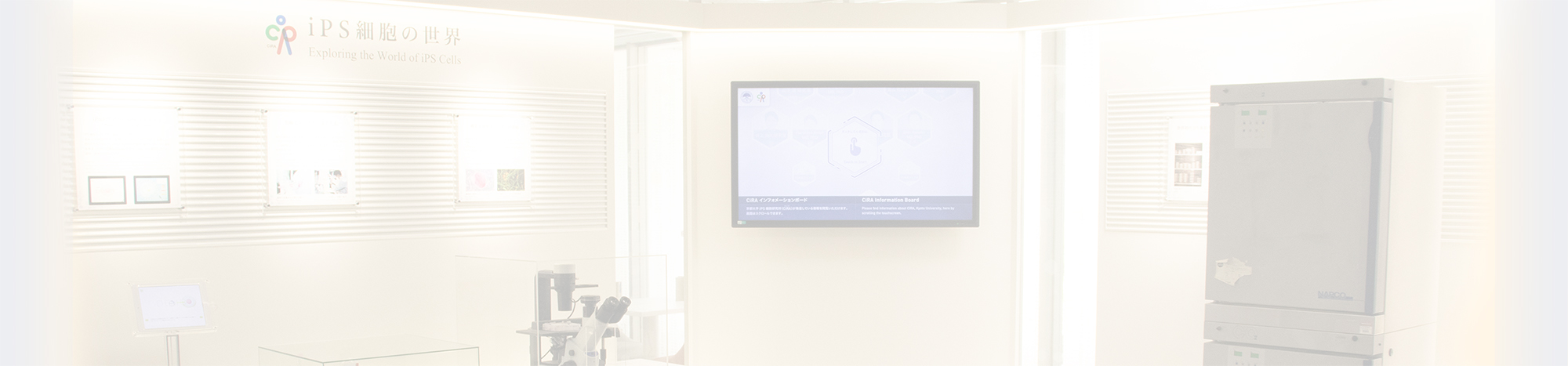
News & Events
News & Events
News
February 24, 2021
Deep learning amyotrophic lateral sclerosis by taking pictures
For many devasting neurological diseases like Alzheimer's disease and amyotrophic lateral sclerosis (ALS), patients are diagnosed only after becoming symptomatic. However, the evidence of memory and muscle loss is seen only after the disease has progressed to irreversible states. Researchers are therefore exploring ways to identify patients well before they become symptomatic, which could allow for wider clinical options.
"If we can catch the disease at the reversible stage, we may reverse and cancel the disease." said CiRA Prof. Haruhisa Inoue, a specialist of ALS research using iPS cells.
Recently, researchers have reported that deep learning could potentially identify individuals at risk for Alzheimer's disease (https://www.nytimes.com/2021/02/01/health/alzheimers-prediction-speech.html). In the new study on ALS seen in Annals of Neurology, Inoue and colleagues show how deep learning can be used to identify indications of ALS, a relentless motor neuron disease, by using iPS cell technology to reprogram patient blood cells into motor neurons, the cells most afflicted by the disease.
Convolutional neural networks were trained using images of motor neurons prepared from the iPS cells of 15 healthy donors and 15 ALS patients. Based on just images of motor neurons, the network could predict with over 0.97 of area under curve (AUC) whether other donors were healthy or ALS patients. On the other hand, conventional machine learning with the same images, focusing on soma size, neurite length, and cell number, presented approximately 0.97 of AUC (see figure).
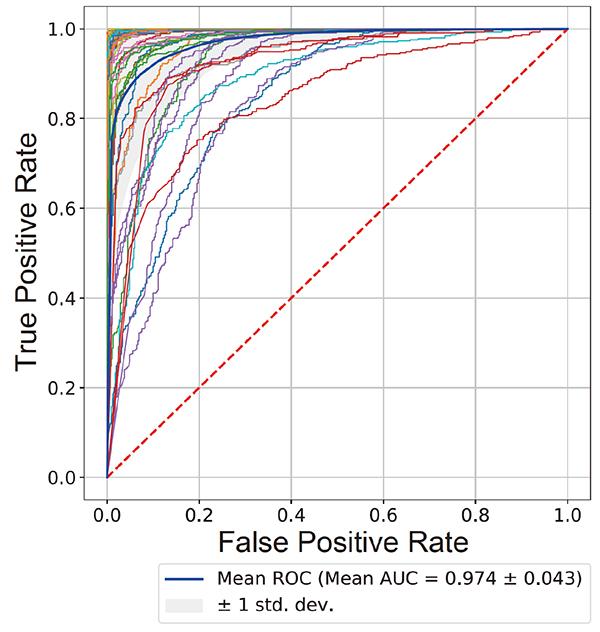
Figure1: Receiver Operating Characteristics (ROC) curve for classifying healthy individuals and ALS patients from 55 test data sets. The average curve is shown by the thick blue line. The red dotted line shows equal true/false positives. The ROC curve shows the accuracy of the model in predicting true and false ALS cases, and Area Under Curve (AUC) is the area underneath the ROC curve. AUC values closer to 1 indicate higher accuracy.
Deep learning as a cell imaging diagnostic tool has made notable inroads for several types of cancers. Inoue is hopeful that the application to ALS will benefit new diagnostics.
"Motor neurons are the primary cells affected by ALS, but these cannot be examined directly. iPS cells allows us to prepare motor neurons with the same genetic background as the patient. Using deep learning to analyze those cells, we may predict ALS at different stages of the disease." he said.
Paper Details
- Journal: Annals of Neurology
- Title: Prediction model of Amyotrophic Lateral Sclerosis by Deep Learning with Patient Induced Pluripotent Stem Cells
- Authors: Keiko Imamura1,2,3, Yuichiro Yada2,3, Yuishin Izumi4, Mitsuya Morita5, Akihiro Kawata6, Takayo Arisato7, Ayako Nagahashi1,2, Takako Enami1,2, Kayoko Tsukita2,3, Hideshi Kawakami8, Masanori Nakagawa9, Ryosuke Takahashi10, Haruhisa Inoue1,2,3,11
- Author Affiliations:
- Medical-risk Avoidance based on iPS Cells Team, RIKEN Center for Advanced Intelligence Project (AIP), Kyoto, 606-8507, Japan
- Center for iPS Cell Research and Application (CiRA), Kyoto University, Kyoto, Japan
- iPSC-based Drug Discovery and Development Team, RIKEN BioResource Research Center (BRC), Kyoto, Japan
- Department of Clinical Neuroscience, Institute of Biomedical Sciences, Tokushima University Graduate School, Tokushima, Japan
- Division of Neurology, Department of Internal Medicine, Jichi Medical University, Tochigi 329-0498, Japan
- Department of Neurology, Tokyo Metropolitan Neurological Hospital, Tokyo 1830042, Japan
- Department of Neurology, National Hospital Organization Minamikyusyu Hospital, Kagoshima, Japan
- Department of Epidemiology, Research Institute for Radiation Biology and Medicine, Hiroshima University, Hiroshima, Japan
- Department of Neurology, Kyoto Prefectural University of Medicine, Kyoto, Japan
- Department of Neurology, Graduate School of Medicine, Kyoto University, Kyoto, Japan
- Institute for Advancement of Clinical and Translational Science (iACT), Kyoto University Hospital, Kyoto, Japan