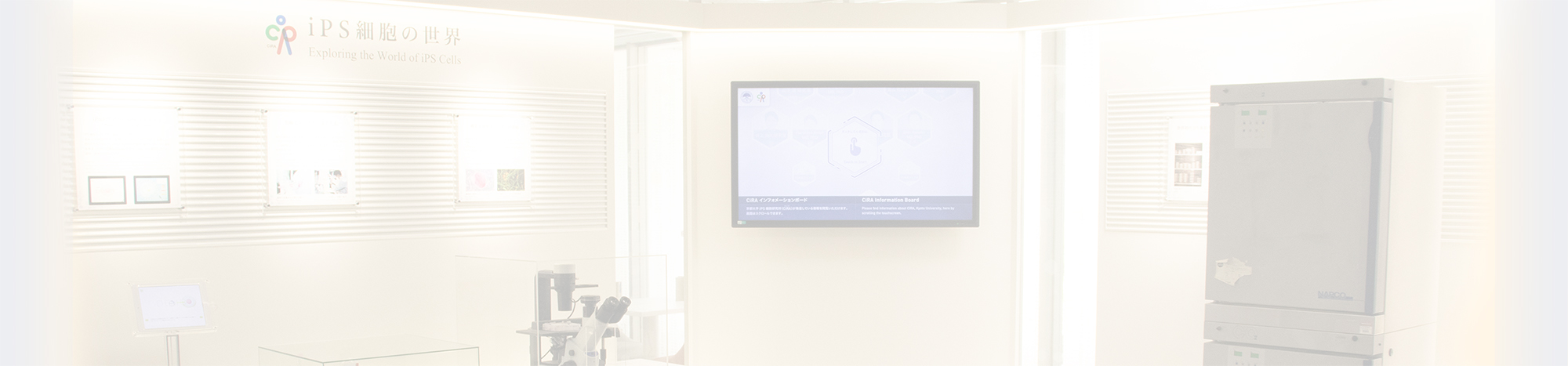
News & Events
News & Events
News
January 19, 2024
Constructing a deep generative approach for functional RNA design
While antisense oligonucleotide and aptamer drugs have been on the market since the 2000s, it was not until the development of SARS-CoV2 mRNA vaccines employed to fight against the COVID-19 pandemic that RNA-based therapeutics attracted the attention of the general public.
In contrast, because of their immense potential-not only for medical applications but for basic biological research and biotechnology-RNA engineering has been on the scientific forefront for decades. As such, there is a tremendous interest in revolutionizing current approaches for designing RNA sequences. Remarkably, there is still no versatile computational platform for functional RNA design. Most existing approaches function by reconstructing specific secondary structures or are restricted to particular types of sequences, such as CRISPR gRNA, mRNA, or specific riboswitches. Since these traditional approaches typically depend on predicting and optimizing RNA secondary structures, their accuracy is inherently constrained by structural prediction and optimization algorithms. A novel approach was thus necessary to avoid these limitations and produce powerful and robust computational methods to construct RNA with desired functions.
The research team aimed to avoid these problems by focusing on RNA families, which are sequence groups with thousands of functional RNAs endowed with identical functions. Even with only a few hundred sequences, multiple sequence alignment can create a consensus secondary structure from which new sequences can be generated. As this computational platform theoretically works with any functional RNA families, the researchers named their deep generative model the RNA family sequence Generator, or RfamGen, which is the world's first deep generative model for functional RNA design.
RfamGen combines two approaches: (1) covariance model and (2) variational autoencoder. The covariance model is a type of statistical framework for RNA alignment and consensus secondary structure that quantitatively evaluates variations of sequence and structure. Meanwhile, the variational autoencoder is a deep generative model with an internal representation called "latent space" to mitigate the complexity associated with exploring the exponentially vast sequence space for the optimization of RNA sequences. By leveraging these two concepts, the researchers generated a system that learns sequence and structural information to explore new RNA designs logically, a feat that has never been done previously.
The team first compared RfamGen, which considers both alignment and secondary structural information, with models accounting for either alignment or secondary structural information, or neither.
For the 18 RNA families tested (each with alignments comprised of at least 10,000 sequences), RfamGen showed a significantly improved ability to generate high-quality RNA sequences. Furthermore, the researchers also tested RfamGen's capabilities when restricted to a limited number of input sequences from which to learn. Despite only being trained on 500 input sequences, RfamGen successfully generated RNA sequences with high scores, thus demonstrating its efficient generative capacity.
The researchers next trained RfamGen using 629 RNA families in total, each with at least 100 sequences from the Rfam database, and found RfamGen performs substantially better compared to other systems. The researchers, furthermore, evaluated how well generated RNA sequences function by randomly synthesizing several RNA sequences generated from training it with a diversity of self-cleavage ribozymes and from random sampling a covariance model. Notably, the sequences generated by RfamGen showed enzymatic activity, while the randomly sampled sequences did not, indicating RfamGen learned important features essential for functionality from the training data.
Lastly, the research team utilized the ligand-dependent self-cleavage activity of the glmS ribozyme as a comparative platform to benchmark generated sequences by RfamGen to natural glmS sequences. They first trained RfamGen using about 500 natural glmS ribozyme sequences and sampled the "latent space" to obtain 1,000 generated sequences. Using a massively parallel assay, they tested these 1,000 generated sequences, 761 natural sequences in the glmS ribozyme family (RF00234), and 100 sequences with kinetic measurements from a previous report. Not only did the team observe the generated sequences to possess a similar distribution of cleavage kinetics as natural sequences, but remarkably found that generated sequences showed higher cleavage rates compared to natural sequences, thus suggesting RfamGen successfully generates high-quality sequences with comparable or higher efficiency than some natural sequences.
The golden age of RNA-based bioengineering is on the horizon. By constructing this deep generative model for functional RNA design, the research team believes RfamGen will be a fundamental driving force to propel RNA biology into a new era and enable discoveries and applications based on RNA.
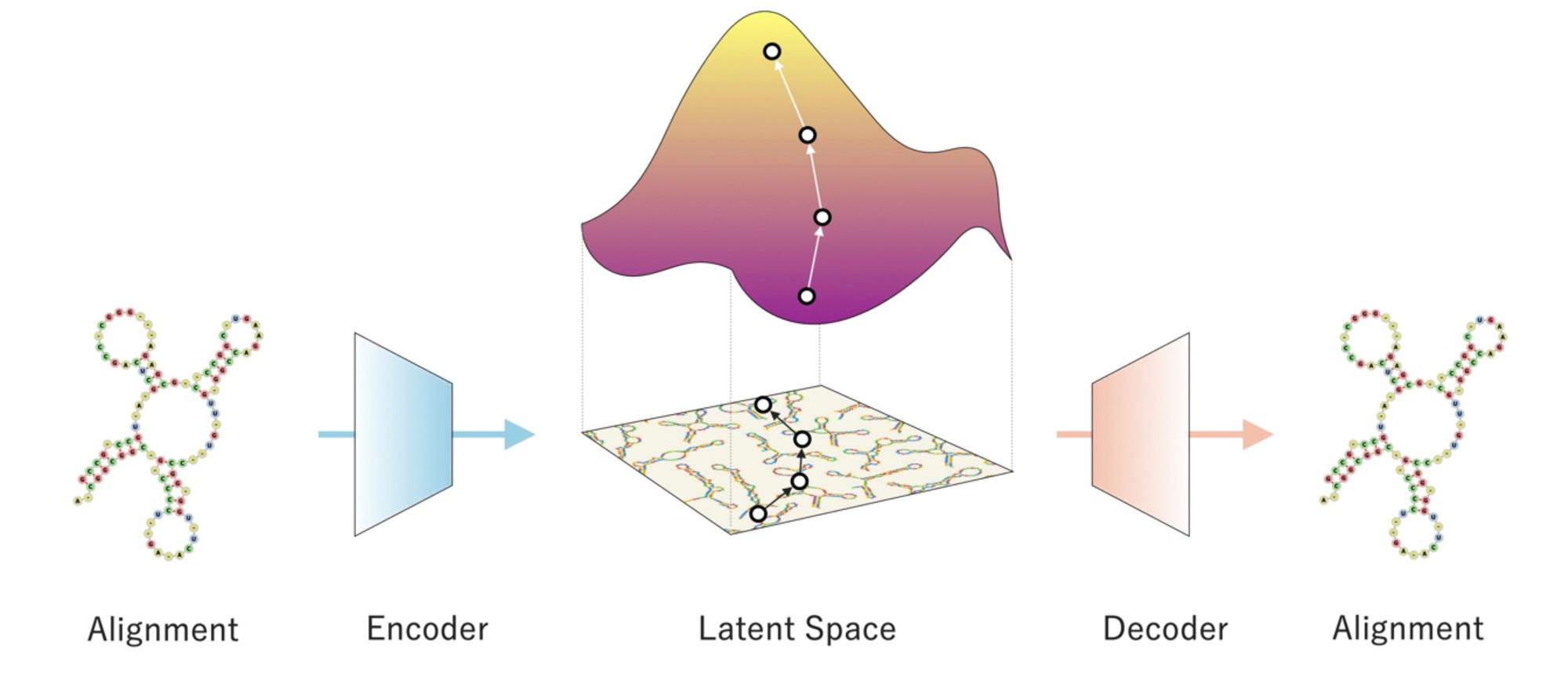
Deep generative model of functional RNA
Paper Details
- Journal: Nature Methods
- Title: Deep generative design of RNA family sequences
- Authors: Shunsuke Sumi1,2,3, Michiaki Hamada3,4,5,*, Hirohide Saito1,*
* : Corresponding authors - Author Affiliations:
- Center for iPS Cell Research and Application (CiRA), Kyoto University
- Graduate School of Medicine, Kyoto University
- Graduate School of Advanced Science and Engineering, Waseda University
- Computational Bio Big-Data Open Innovation Laboratory (CBBD-OIL), National Institute of Advanced Industrial Science and Technology (AIST)
- Graduate School of Medicine, Nippon Medical School