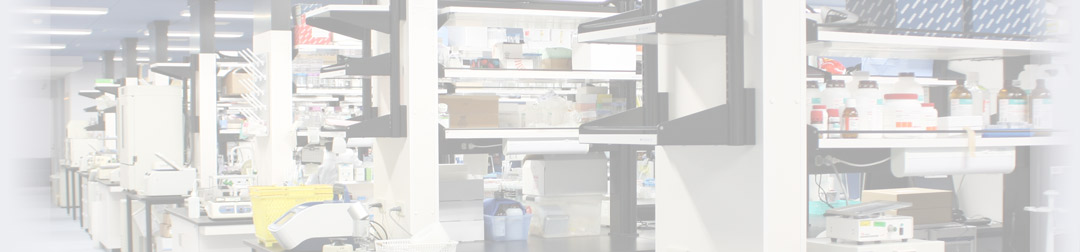
Research Activities
Research Activities
Publications
August 29, 2019
Machine learning builds a 3D mouse embryo model
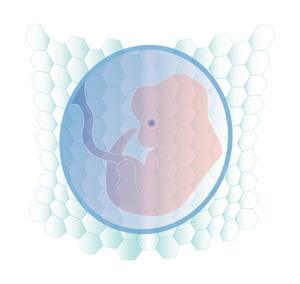
The possibility of using stem cells to form 3D organs and tissues is expected to revolutionize regenerative medicine. One option for predicting how such complex 3D structures emerge is by growing them in the lab, but at high cost. Alternatively, computer models can be used to predict their growth from a minimum set of data. A new study by scientists at CiRA reports a computer algorithm called SPRESSO, or SPatial REconstruction by Stochastic SOM, that shows the location of cells in a gastrulating mouse embryo. SPRESSO reliably predicts the overall organization of this early embryonic structure, which goes on to form the entire mouse body, by examining just 18 of more than 20,000 genes.
Genes are the blueprint of our bodies. Their dynamic expression results in cells duplicating, morphing, or interacting with other cells to form higher level structures like tissues and organs. Somehow, spatial information is acquired from these gene expressions so that our hands are at the end of our arms and not at our shoulders, but which genes are most crucial for this spatial information remains elusive.
Scientists have already measured the expression of more than 20,000 genes from 41 cell samples of the gastrulating mouse embryo. Prof. Wataru Fujibuchi, who led the study, used these samples and genes as input for SPRESSO.
"20,000 genes is too big a number for practical simulations. Our goal was to reduce the list to only those genes that regulate spatial patterns," he says.
Thus, one of SPRESSO's goal was to identify these genes by using stochastic self-organizing maps.
"Stochastic self-organizing maps is an algorithm that uses unsupervised learning. It was first applied to study neural networks but now used to study economics, geography and other fields," explains Fujibuchi.
To more accurately recreate the structural details of a three-dimensional embryo based on available gene expression data, the researchers applied a modification of such maps known as stochastic self-organizing map clustering.
With this adjustment, Fujibuchi's team took gene ontology lists from public bioinformatics databases and was able to reduce the list to just 18 specific genes. Analyzing the expression levels of these genes could computationally place the 41 samples in their proper position of a gastrulating embryo, which starts to form the three principal germ layers that give rise to all future tissues and organs of the developing embryo.
Notably, all 18 genes were recognized as having a role in morphogenesis and differentiation, providing what Fujibuchi describes as a gene set of "spatial discriminators".
"Focusing on the expression of spatial discriminators could be sufficient for simulating 3D structures, significantly reducing laboratory costs," he says.
Further analysis found that the exclusion of one gene, Inhibitor of DNA binding 2 (ID2), from the list of 18 genes severely compromised the simulation.
The findings suggest that a remarkably complex system can be simplified dramatically for the study of what is life's beginnings.
"Without spatial information we could reconstruct the architecture of the gastrulation-stage embryo based on gene expression data alone with 100% accuracy, which is astonishing. Further investigations of spatial discriminator genes will enhance our understanding of coordinated gene expressions in 3D self-organization to produce living tissues," says Fujibuchi.
Paper Details
- Journal: Scientific Reports
- Title: Novel computational model of gastrula morphogenesis to identify spatial discriminator genes by self-organizing map (SOM) clustering
- Authors: Tomoya Mori1, Haruka Takaoka2, Junko Yamane1, Cantas Alev1, and Wataru Fujibuchi1
- Author Affiliations:
- Center for iPS Cell Research and Application, Kyoto University, Kyoto, Japan
- 2Department of Life Science and Informatics, Faculty of Engineering, Maebashi Institute of Technology, Maebashi City, Gunma, Japan